DeepMind’s new AI promises world’s most accurate 10-day weather forecasts
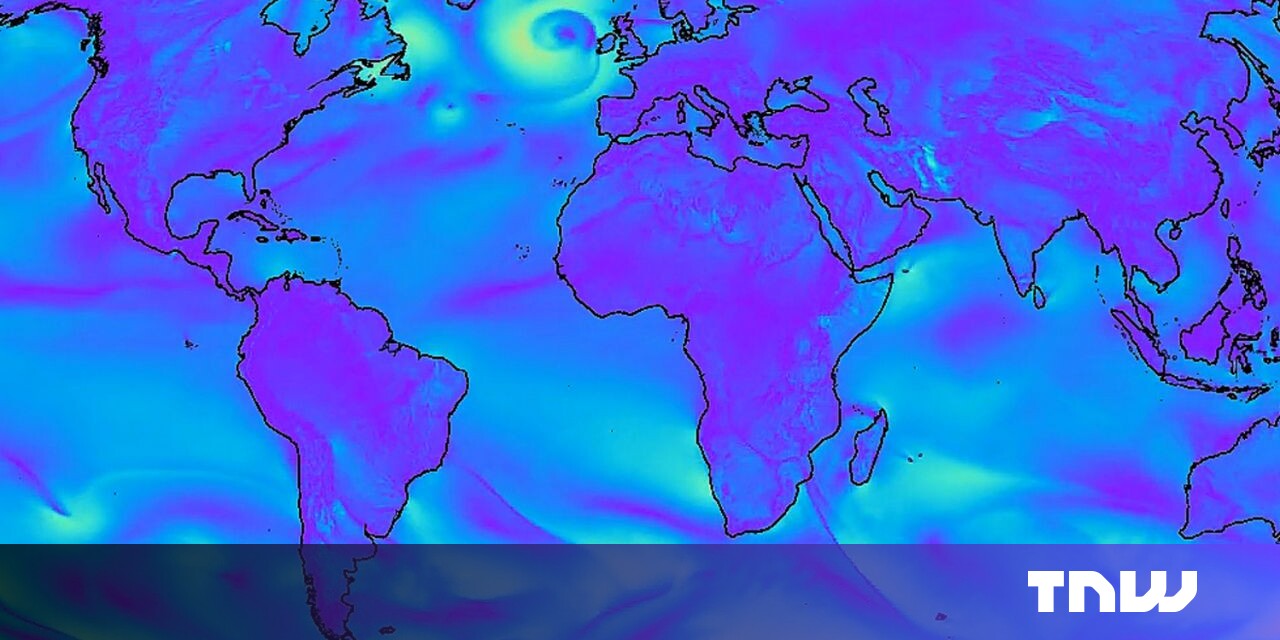
[ad_1]
A new AI model Google DeepMind is the world’s most accurate 10-day global weather forecasting system, according to the London-based lab.
Named GraphCast, the model promises medium-range weather forecasts of “unprecedented accuracy.” In research published today, GraphCast was found to be more precise and faster than the industry gold standard for weather simulation, the High-Resolution Forecast (HRES).
The system also predicted extreme weather from a more distant future than was previously possible. These insights were analysed by the European Centre for Medium-Range Weather Forecasts (ECMWF), an intergovernmental organisation that produces the HRES.
A live version of Graphcast was deployed on the ECMWF website. In September, the system accurately predicted that Hurricane Lee would make landfall in Nova Scotia around nine days in advance.
In contrast, traditional forecasting methods only spotlighted Nova Scotia around six days ahead of time. They also provided less consistent predictions of the time and location of landfall.
Intriguingly, GraphCast can identify dangerous weather events without being trained to find them. After integrating a simple cyclone tracker, the model predicted cyclone movement more accurately than the HRES method.
Such data could save lives and livelihoods. As the climate becomes more extreme and unpredictable, fast and accurate forecasts will provide increasingly vital insights for disaster planning.
Matthew Chantry, a machine learning coordinator at the ECMWF, believes his industry has reached a monumental turning point.
“There’s probably more work to be done to create reliable operational products, but this is likely the beginning of a revolution,” Chantry said at a press briefing.
Meteorological organisations, he added, had previously expected AI to be most useful when merged with physics. Recent breakthroughs, however, show machine learning can also directly forecast weather.
How GraphCast works
Conventional weather forecasts are based on intricate physics equations. These are then adapted into algorithms that can run on supercomputers.
The process can be painstaking. It also requires specialist knowledge and vast computing resources.
GraphCast harnesses a different technique. The model combines machine learning with Graph Neural Networks (GNNs), an architecture that’s adept at processing spatially structured data.
To learn the causes and effects that determine weather changes, the system was trained on decades of weather information.
Traditional approaches are also incorporated. The ECMWF supplied Graphcare with training data from around 40 years of weather reanalysis, which encompassed monitoring from satellites, radars and weather stations.
When there are gaps in these observations, traditional physics-based prediction methods fill them in. The result is a detailed history of global weather. GraphCast uses these lessons from the past to predict the future.
The forecasts cover the entire surface of the world. A single weather state is predicted at the high resolution of 0.25-degree latitude/longitude. (roughly 28 km × 28 km at the equator. To put that into perspective, that covers more than a million grid points across the Earth’s surface.
At each grid point, the model predicts five Earth-surface variables that combine temperature, wind speed and direction, and mean sea-level pressure – and six atmospheric variables at each of 37 levels of altitude, including specific humidity, wind speed and direction, and temperature.
The forecasting model is also highly efficient. Making 10-day forecasts with GraphCast takes less than a minute on a single Google TPU v4 machine. For comparison, a 10-day forecast using a conventional approach, such as HRES, can take hours of computation in a supercomputer with hundreds of machines.
[ad_2]
Source link